3 Common Lead Scoring Pitfalls: Symptoms and Solutions
In B2B marketing lead scoring is certainly not a new concept and has been around for awhile in one form or another, yet still companies struggle to truly realize the benefits and conversion rates continue to drag. Lead scoring promises true marketing and sales alignment that will make sales more productive by focusing them on ideal targets and/or leads furthest through the buying cycle - so why are lead scoring models still not prioritizing the right leads?
We think it could come down to a few common pitfalls that we see often, but that can be rectified relatively quickly.
Pitfall #1: Sending leads to SDRs/Sales too early
Often marketers are nervous to “hold leads back from sales” because they think that they may be holding back good leads from their team or simply because they want to show they are producing MQLs. This battle seems to have been raging since lead scoring began and is something that can be easily resolved.
Symptoms:
- Your SDRs are struggling to work all their leads
- Your conversion rate from MQL/AQL to Sales Qualified is low (below 20%)
- Conversion is taking a long time (i.e., weeks or months instead of days)
Solution:
Consider increasing your threshold for MQLs, thereby reducing the number of MQLs (sorry!). This may sound scary if marketing has an MQL target but MQLs that get recycled or stale often get lost in the mix and not targeted by marketing. Also, it is common place (we often argue against this) to stop nurturing leads that SDRs are working. If we are sending the lead to the SDR too early, suppressing from marketing and the SDR is being ignored - what do we expect to happen? Wouldn’t it be better to keep that lead back a while longer, promote interesting, targeted and thoughtful content to the lead, “warm it up”, build the interest score and then send to sales when it is truly ready?
For those worried that sales may not get “enough leads” to work, you can create an Engaged (but not MQL) view for sales that allows them to pull leads forward. This is essentially a human MQL model which of course comes with the positives and negatives of human intuition. However, typically leads that get pulled forward by SDRs convert at a higher rate and we ensure these leads get stamped as an MQL, but with the grade “SDR Pulled”. This allows you to review these leads separately to compare conversion rates and understand the commonalities of highly converting “SDR Pulled” leads that we can build back into the automated model.
Pitfall #2: Not scoring specific behaviors correctly
It is very easy to give different behavior types a score. Webinar attendance = 20 points, Downloaded Whitepaper = 15 points, etc. However, sometimes this is too simplistic. Not all content within a specific content type are born equal. Someone downloading your whitepaper “Three 2017 Trends in Industry X” indicates a very different level of interest than “A Buyer's Guide to Product X.” However far too often these activities are scored the same as they are both the same content type. In this case, how are we prioritizing leads based on their stage in the buying cycle? I would argue we are falling short.
Symptoms:
- You’re not prioritizing behaviors accurately, and therefore finding that SDRs or sales are recycling leads too early and complaining that the lead wasn’t interested.
- SDRs or sales are cherry-picking leads who have had buying behavior but haven’t MQLed, like the SDR pulled scenario explained above.
Solution:
We suggest companies score specific content and events differently based on the offer. This isn’t as difficult as it sounds. One method we often implement is to give all engagements a base score (e.g. webinar attendance = +10). Then in the webinar program template we will have a score boost smart campaign for +15. If this is a late stage webinar we will activate it, if not we simply delete it. Another way you can do this is by adding a code to your program name:“YEAR-Program-Name-LS”. The “-LS” indicates Late Stage and global scoring rules will track a success in any program with the code in it and score an extra boost.
Pitfall #3: Not utilizing a lead scoring matrix effectively
Often we see MQL or AQL models that either combine behavior and fit score, or just use behavior score. If you want true marketing and sales alignment, you not only want to send them interested leads, but also leads that fit your target buying profile. Now that we are in the days of ABM, this is increasingly important. Sending just engaged leads or combining the score and implementing a threshold isn’t going to cut it.
Also, we see cases where only leads with specific attributes get sent to sales (i.e. Revenue has to be over $100m). This is all well and good if 100% of our leads have the revenue field complete, but often you receive leads without this information.
Symptoms:
- You’re not using a matrix effectively and therefore finding that many of your MQLs are becoming disqualified, possibly impacting how SDRs/sales follows up/trusts all leads from marketing
- You are putting in too rigid of demographic requirements based on a few fields alone. If they aren’t being enriched, you may be missing out on sending a great lead over to sales.
Solution:
This is where the simple, tried and tested lead scoring matrix really is effective. By matching certain levels of behavior score and fit score you can prioritize leads that are a great fit by sending them to sales when they have less than average engagement. You can also prioritize leads with tons of engagement, but may not be the ideal fit. Remember a lead may have a low fit score because it’s data needs enrichment.
This allows you to send a mix of leads to sales that prioritizes BOTH target profile and engagement. The real beauty from this comes from continuous refinement of the matrix levels with inputs from quantitative feedback from conversion reports and qualitative data from developing a SDR feedback loop.
This can also be improved by moving from your typical demographic scoring to a predictive vendor like Radius, Lattice or Infer. However, the matrix concept stays the same just the input into the fit score is modified.
Example of a scoring matrix:
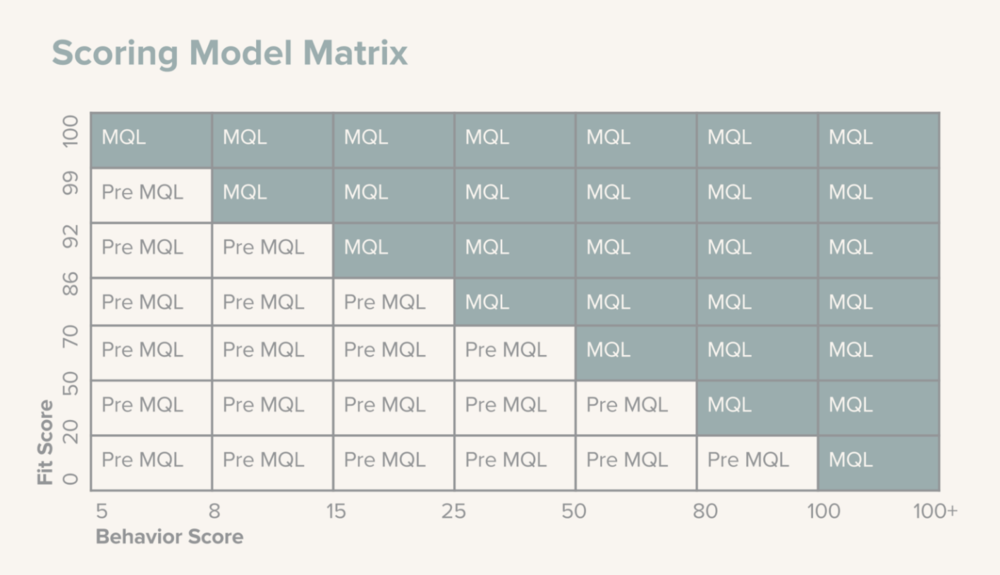
Lead Scoring can feel like an uphill battle with too many variables to count, but taking a step back and refining certain aspects can be a real win for sales and marketing alignment and sales effectiveness.
If you are looking to improve your model or create a new one, check out our Lead Scoring MQL Simulator.